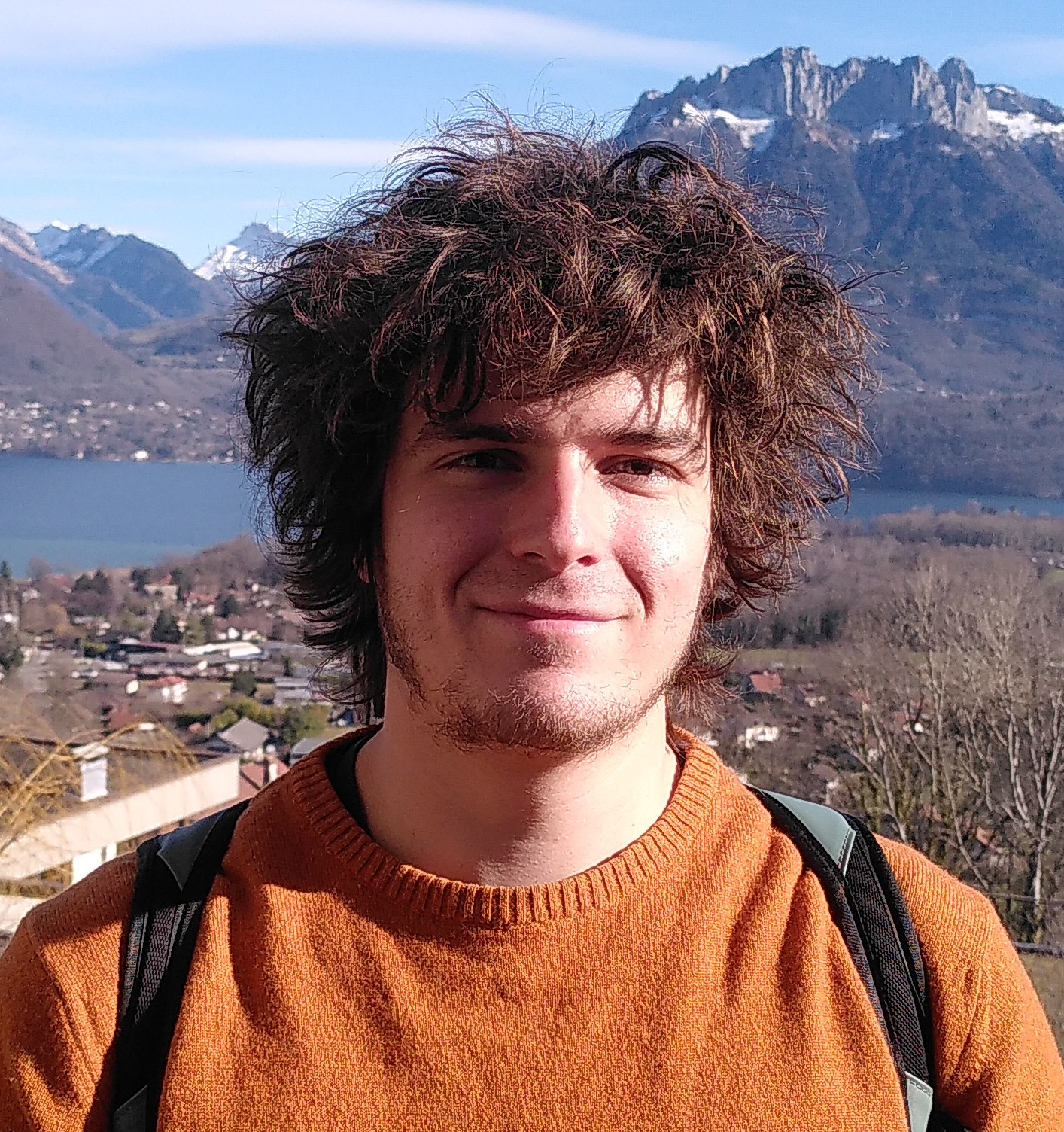
Axel Marmoret
Assistant Professor at IMT Atlantique (BRAIn team)
PhD Graduate in Signal Processing, Computer Science Engineer
International Journals
Accepted
Barwise Music Structure Analysis with the Correlation Block Matching Segmentation Algorithm.
Axel Marmoret, Jérémy E. Cohen, Frédéric Bimbot. Transactions of the International Society for Music Information Retrieval, 6(1), 167--185. DOI: https://doi.org/10.5334/tismir.167.@article{marmoret2023barwise, author = {Marmoret, Axel and Cohen, Jérémy E. and Bimbot, Frédéric}, doi = {10.5334/tismir.167}, journal = {Transactions of the International Society for Music Information Retrieval}, keyword = {en_US}, volume = {6}, number = {1}, pages = {167--185}, title = {Barwise Music Structure Analysis with the Correlation Block-Matching Segmentation Algorithm}, month = {Nov}, year = {2023}}
Abstract: Music Structure Analysis (MSA) is a Music Information Retrieval task consisting of representing a song in a simplified, organized manner by breaking it down into sections typically corresponding to 'chorus', 'verse', 'solo', etc. In this work, we extend an MSA algorithm called the Correlation Block-Matching (CBM) algorithm introduced by (Marmoret et al., 2020, 2022b). The CBM algorithm is a dynamic programming algorithm that segments self-similarity matrices, which are a standard description used in MSA and in numerous other applications. In this work, self-similarity matrices are computed from the feature representation of an audio signal and time is sampled at the bar-scale. This study examines three different standard similarity functions for the computation of self-similarity matrices. Results show that, in optimal conditions, the proposed algorithm achieves a level of performance which is competitive with supervised state-of-the-art methods while only requiring knowledge of bar positions. In addition, the algorithm is made open-source and is highly customizable.
International Conferences
Accepted
Convolutive Block-Matching Segmentation Algorithm with Application to Music Structure Analysis.
Axel Marmoret, Jérémy Cohen, Frédéric Bimbot. IEEE Workshop on Applications of Signal Processing to Audio and Acoustics, Mohonk Mountain House, New Paltz, NY, USA, Oct 22-25, 2023.@inproceedings{marmoret2023convolutive, title={Convolutive Block-Matching Segmentation Algorithm with Application to Music Structure Analysis}, author={Marmoret, Axel and Cohen, J{\'e}r{\'e}my E and Bimbot, Fr{\'e}d{\'e}ric}, booktitle={WASPAA}, year={2023}}
Abstract: Music Structure Analysis (MSA) consists of representing a song in sections (such as 'chorus', 'verse', 'solo' etc), and can be seen as the retrieval of a simplified organization of the song. This work presents a new algorithm, called Convolutive Block-Matching (CBM) algorithm, devoted to MSA. In particular, the CBM algorithm is a dynamic programming algorithm, applying on autosimilarity matrices, a standard tool in MSA. In this work, autosimilarity matrices are computed from the feature representation of an audio signal, and time is sampled on the barscale. We study three different similarity functions for the computation of autosimilarity matrices. We report that the proposed algorithm achieves a level of performance competitive to that of supervised state-of-the-art methods on 3 among 4 metrics, while being fully unsupervised.
Barwise Compression Schemes for Audio-Based Music Structure Analysis.
Axel Marmoret, Jérémy Cohen, Frédéric Bimbot. SMC 2022 - 19th Sound and Music Computing Conference, Jun 2022, Saint-Etienne, France.@inproceedings{Barwise Compression Schemes for Audio-Based Music Structure Analysis}, author={Marmoret, Axel and Cohen, J{\'e}r{\'e}my E and Bimbot, Fr{\'e}d{\'e}ric}, booktitle={{Proceedings of the 19th Sound and Music Computing Conference}}, year={2022}, doi= {10.5281/zenodo.6822204}}
Abstract: Music Structure Analysis (MSA) consists in segmenting a music piece in several distinct sections. We approach MSA within a compression framework, under the hypothesis that the structure is more easily revealed by a simplified representation of the original content of the song. More specifically, under the hypothesis that MSA is correlated with similarities occurring at the bar scale, this article introduces the use of linear and non-linear compression schemes on barwise audio signals. Compressed representations capture the most salient components of the different bars in the song and are then used to infer the song structure using a dynamic programming algorithm. This work explores both low-rank approximation models such as Principal Component Analysis or Nonnegative Matrix Factorization and ``piece-specific'' Auto-Encoding Neural Networks, with the objective to learn latent representations specific to a given song. Such approaches do not rely on supervision nor annotations, which are well-known to be tedious to collect and possibly ambiguous in MSA description. In our experiments, several unsupervised compression schemes achieve a level of performance comparable to that of state-of-the-art supervised methods (for 3s tolerance) on the RWC-Pop dataset, showcasing the importance of the barwise compression processing for MSA.
Semi-Supervised Convolutive NMF for Automatic Piano Transcription.
Haoran Wu, Axel Marmoret, Jérémy Cohen. SMC 2022 - 19th Sound and Music Computing Conference, Jun 2022, Saint-Etienne, France.@inproceedings{wu2022semi, title={Semi-Supervised Convolutive NMF for Automatic Music Transcription}, author={Wu, Haoran and Marmoret, Axel and Cohen, J{\'e}r{\'e}my E}, booktitle={{Proceedings of the 19th Sound and Music Computing Conference}}, year={2022}, doi= {10.5281/zenodo.6822204}}
Abstract: Automatic Music Transcription, which consists in transforming an audio recording of a musical performance into symbolic format, remains a difficult Music Information Retrieval task. In this work, which focuses on piano transcription, we propose a semi-supervised approach using low-rank matrix factorization techniques, in particular Convolutive Nonnegative Matrix Factorization. In the semi-supervised setting, only a single recording of each individual notes is required. We show on the MAPS dataset that the proposed semi-supervised CNMF method performs better than state-of-the-art low-rank factorization techniques and a little worse than supervised deep learning state-of-the-art methods, while however suffering from generalization issues.
Uncovering Audio Patterns in Music with Nonnegative Tucker Decomposition for Structural Segmentation.
Axel Marmoret, Jérémy Cohen, Nancy Bertin, Frédéric Bimbot. ISMIR 2020 - 21st International Society for Music Information Retrieval, Oct 2020, Montréal (Online), Canada.@inproceedings{marmoret2020uncovering, title={Uncovering Audio Patterns in Music with Nonnegative Tucker Decomposition for Structural Segmentation}, author={Marmoret, Axel and Cohen, J{\'e}r{\'e}my and Bertin, Nancy and Bimbot, Fr{\'e}d{\'e}ric}, booktitle={ISMIR 2020-21st International Society for Music Information Retrieval}, year={2020}}
Abstract: Recent work has proposed the use of tensor decomposition to model repetitions and to separate tracks in loop-based electronic music. The present work investigates further on the ability of Nonnegative Tucker Decompositon (NTD) to uncover musical patterns and structure in pop songs in their audio form. Exploiting the fact that NTD tends to express the content of bars as linear combinations of a few patterns, we illustrate the ability of the decomposition to capture and single out repeated motifs in the corresponding compressed space, which can be interpreted from a musical viewpoint. The resulting features also turn out to be efficient for structural segmentation, leading to experimental results on the RWC Pop data set which are potentially challenging state-of-the-art approaches that rely on extensive example-based learning schemes.
National Conferences
Accepted
Nonnegative Tucker Decomposition with Beta-divergence for Music Structure Analysis of audio signals.
Axel Marmoret, Florian Voorwinden, Valentin Leplat, Jérémy E Cohen, Frédéric Bimbot. GRETSI 2022: XXVIIIe Colloque, Sep 2022, Nancy, France.@inproceedings{marmoret2022nonnegative, title={Nonnegative Tucker Decomposition with Beta-divergence for Music Structure Analysis of audio signals}, author={Marmoret, Axel and Voorwinden, Florian and Leplat, Valentin and Cohen, J{\'e}r{\'e}my E and Bimbot, Fr{\'e}d{\'e}ric}, booktitle={{GRETSI 2022: XXVIIIe Colloque}}, year={2022}, organization={GRETSI}}
Abstract: Nonnegative Tucker Decomposition (NTD), a tensor decomposition model, has received increased interest in the recent years because of its ability to blindly extract meaningful patterns in tensor data. Nevertheless, existing algorithms to compute NTD are mostly designed for the Euclidean loss. On the other hand, NTD has recently proven to be a powerful tool in Music Information Retrieval. This work proposes a Multiplicative Updates algorithm to compute NTD with the beta-divergence loss, often considered a better loss for audio processing. We notably show how to implement efficiently the multiplicative rules using tensor algebra, a naive approach being intractable. Finally, we show on a Music Structure Analysis task that unsupervised NTD fitted with beta-divergence loss outperforms earlier results obtained with the Euclidean loss.
Thesis
Unsupervised Machine Learning Paradigms for the Representation of Music Similarity and Structure.
Axel Marmoret. PhD thesis in Signal Processing, Université Rennes 1. Under the supervision of Jérémy Cohen and Frédéric Bimbot, with the additional help of Nancy Bertin and Simon Leglaive.@phdthesis{marmoret2022unsupervised, title={Unsupervised Machine Learning Paradigms for the Representation of Music Similarity and Structure.}, author={Marmoret, Axel}, year={2022}, school={Universit{\'e} Rennes 1}}
Abstract: Musical structure, defined as a simplified representation of the organization of a song, is an important musicological concept, but hard to automatically estimate. This thesis presents new methods to automatically estimate the structural segmentation of a song, focusing the study of music at the barscale. By developing a new segmentation algorithm (called ``CBM'') and by comparing several unsupervised compression schemes (from linear and multilinear algebra to neural networks), paradigms introduced in this thesis result in segmentation performance outperforming those of the unsupervised State-of-the-Art methods and almost similar with those of the global State-of-the-Art, obtained with supervised machine learning algorithms. In particular, as the methods described in this thesis are unsupervised, the estimation do not rely on annotated data, lowering the bias in the estimates related to ambiguity and subjectivity (inherent to musical structure) while limiting the loss in performance compared to the best supervised methods. In addition, some of the methods studied in this thesis (in particular Nonnegative Tucker Decomposition) allow to extract automatically interpretable parts of a song which may be used for other task than the estimation of structure, and participate in the development of interpretable machine and deep learning algorithms, which is a major field of research nowadays.
Multi-Channel Automatic Music Transcription Using Tensor Algebra.
Axel Marmoret. Master's thesis. Under the supervision of Nancy Bertin and Jérémy Cohen. arXiv preprint arXiv:2107.11250.@article{marmoret2019multi, title={Multi-Channel Automatic Music Transcription Using Tensor Algebra}, author={Marmoret, Axel and Bertin, Nancy and Cohen, J{\'e}r{\'e}my}, journal={arXiv preprint arXiv:2107.11250}, year={2019}}
Abstract: Music is an art, perceived in unique ways by every listener, coming from acoustic signals. In the meantime, standards as musical scores exist to describe it. Even if humans can make this transcription, it is costly in terms of time and efforts, even more with the explosion of information consecutively to the rise of the Internet. In that sense, researches are driven in the direction of Automatic Music Transcription. While this task is considered solved in the case of single notes, it is still open when notes superpose themselves, forming chords. This report aims at developing some of the existing techniques towards Music Transcription, particularly matrix factorization, and introducing the concept of multi-channel automatic music transcription. This concept will be explored with mathematical objects called tensors.
Pre-prints
Polytopic Analysis of Music.
Axel Marmoret, Jérémy Cohen, Frédéric Bimbot. arXiv preprint arXiv:2212.11054..@article{marmoret2022polytopic, title={Polytopic Analysis of Music}, author={Marmoret, Axel and Cohen, J{\'e}r{\'e}my E and Bibmot, Fr{\'e}d{\'e}ric}, journal={arXiv preprint arXiv:2212.11054}, year={2022}}
Abstract: Structural segmentation of music refers to the task of finding a symbolic representation of the organisation of a song, reducing the musical flow to a partition of non-overlapping segments. Under this definition, the musical structure may not be unique, and may even be ambiguous. One way to resolve that ambiguity is to see this task as a compression process, and to consider the musical structure as the optimization of a given compression criteria. In that viewpoint, C. Guichaoua developed a compression-driven model for retrieving the musical structure, based on the "System and Contrast" model, and on polytopes, which are extension of nhypercubes. We present this model, which we call "polytopic analysis of music", along with a new opensource dedicated toolbox called MusicOnPolytopes (in Python). This model is also extended to the use of the Tonnetz as a relation system. Structural segmentation experiments are conducted on the RWC Pop dataset. Results show improvements compared to the previous ones, presented by C. Guichaoua.